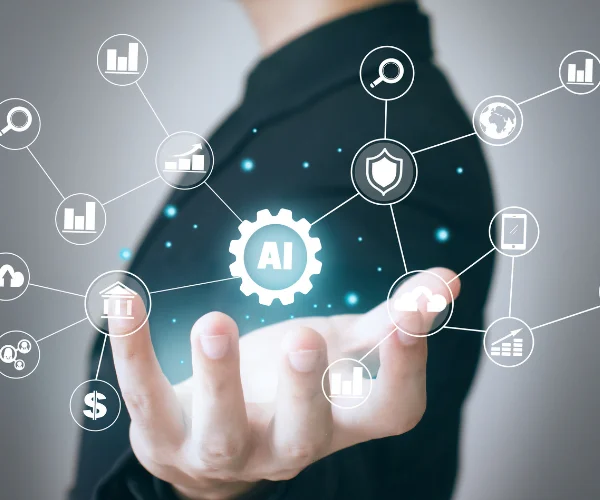
In an era where information is omnipresent, the discernment of bias within news articles and media outlets has become paramount. With this in mind, how can we trust data analysts and artificial intelligence (AI) systems to determine what is genuinely accurate? This question is particularly pertinent in the operations of Biasly.
The Human-AI Relationship in Bias Detection
Through its proprietary Bias Meter, Biasly operates under a dichotomy of human analysis and artificial intelligence. Our AI algorithm, built upon a deep neural network of natural language processing, is adept at excavating entities within news articles, discerning political sentiments, and amalgamating them with party policy sentiments to compute a Bias Score.
However, we are not purely AI-driven. The role of Biasly’s diverse staff is indispensable in creating a balanced and nonpartisan perspective. We dissect content, sentence by sentence, to discern whether excerpts harbor a bias or dwell in neutrality. This constructive collaboration between humans and machines permits a layered, meticulous approach to identifying and quantifying bias.
The Unbiased Nature of Algorithms: Myth or Reality?
Contrary to the notion that AI algorithms are devoid of bias, considering they lack inherent beliefs or political inclinations, the reality can be slightly divergent. AI systems, including the Bias Meter, are trained on large volumes of data that, unfortunately, can emanate from biased environments or be imbued with human prejudices. Consequently, the pivotal question morphs into how Biasly ensures its AI system does not reflect the personal preferences within the articles it evaluates.
The Checks and Balances Within Biasly
Biasly employs a systematic approach to refine, adjust, and enhance the Bias Meter continually. The equipoise between a diverse group of data analysts and the machine learning algorithm fortifies how biases are detected and evaluated. The spectrum of analysts, stretching across all political and ideological aisles, serves as a built-in check against potential biases that might inadvertently seep into the AI’s analytical processes.
Furthermore, the algorithm is persistently evolving, learning not only from the new data it encounters but also from the corrections and inputs from human analysts. This dynamic, self-improving characteristic of the Bias Meter facilitates its accuracy and reliability.
Our Seven Rating Metrics Used by Analysts
To elucidate the technical yet meticulous bias rating methodology employed by Biasly, we will delve into the seven rating metrics applied during the bias analysis process:
- Tone: The measurement of an author’s opinion and political leaning, scrutinizing whether they communicate positively or negatively about topics, politicians, or policies. The Tone metric seeks to recognize and rate the magnitude of any bias exhibited through an author’s expressive style.
- Tendency: Investigating how consistently the identified tone appears throughout the article. The Tendency metric evaluates the persistent occurrence of bias throughout the text, providing insight into its pervasiveness.
- Diction: This metric evaluates an author’s word choice, especially the utilization of extremes and trigger words that could incite specific emotions or actions from the reader. The Diction acts as a gauge to measure the extremity of language used, evaluating whether the terminology affirms or rejects stances.
- Author Check: It is always important to thoroughly investigate the author’s political affiliation, ideology, and potential agenda. This measure scrutinizes an author’s public profiles and previous works to discern any prevalent leanings or biases that might influence their writing.
- Selection/Omission: Identifying whether the author strategically omits or includes specific facts to steer reader perception. The Selection/Omission metric scrutinizes the balance and fairness of presented facts, ensuring no omissions of critical information to affirm a bias.
- Expediency Bias: Analyzing first impressions from the headline, images, and summary, the Expediency Bias metric evaluates the initial emotional impact and seeks traces of click-bait biases that may mislead readers.
- Accuracy: A pivotal metric that scrutinizes the validity of sources and claims within the article. Accuracy diligently examines a source’s truthfulness, reliability, and sufficiency, ensuring that facts are correct and adequately supported by credible references.
Evaluating Political Party Policy Stances
Understanding party policy stances becomes pivotal when navigating the complex political spectrum. Recognizing this reality, Biasly embarks on a meticulous journey of evaluating these stances, ensuring the Bias Meter effectively tunes itself to the nuanced preferences and inclinations inherent within the diverse media landscape. We intertwine polling results, explicit party stances, and Congressional voting patterns to construct a coherent image of the predominant political predilections of conservatives and liberals.
Biasly’s methodology distills this information into understandable and quantifiable metrics. We analyze the stances of prominent political figures, Democratic and Republican, on myriad political issues. These foundational stances for understanding bias extrapolate from the collective opinions of each party’s constituents and representatives. The data we gather is a crucial component in the analytical processes of the Bias Meter.
Biasly ensures that the algorithm does not merely utilize this data in a vacuum but integrates it to understand the subtle and often implicit biases that may permeate a news article or media report. The evaluation of party stances does not simply stop at understanding; it extends toward critically examining how they are portrayed, manipulated, or obfuscated within media narratives.
Moreover, scrutinizing policy stances involves examining how media discourse communicates, frames, and perceives these policies. This dimensional analysis helps discern explicit and implicit biases that color a reader’s perception. It also triangulates the political parties’ direct statements, their members’ voting behavior, and the prevailing public sentiment and policy impact. Doing so allows news consumers to understand, gauge, and quantify media bias more effectively.
In understanding and analyzing these policy stances, Biasly empowers itself to refine the functionality of the Bias Meter, enhancing its ability to navigate the intricate, multifaceted, and often obscured biases that might emerge within various media narratives.
Real-Time Implications and Impact
Biasly does not merely guard against partisanship in the intricate landscape of political media. On a larger scale, it proactively engages in the real-time processing and meticulous fetching of news, scrupulously offering users multifaceted insights. This benefit, which robustly fortifies media literacy, also enhances the understanding and knowledge of readers regarding global events and complex political narratives.
The Future: A Bias-Free Horizon?
While Biasly offers a robust mechanism in the ongoing struggle against bias in media and political narratives, the journey towards an entirely bias-free horizon is too good to be true. Regardless, when continually scrutinized, refined, and held accountable, data analysts and AI collaboration offer a formidable tool in this journey, sculpting a path toward more balanced and unbiased media consumption.
Biasly functions as a sentinel of media integrity, leveraging human oversight and artificial intelligence’s harmonious interplay to navigate the multifaceted complications of media bias. Trust in such a system burgeons from its technological prowess and unwavering commitment to scrutiny, transparency, and perpetual evolution in its quest to sift through bias and arrive at the most honest, undiluted truth possible.